In the ever-evolving world, Feature Engineering in AI stands as a pivotal practice, influencing a multitude of domains. In machine learning, natural language processing, data analytics, and time series analysis, the significance of feature engineering is irrefutable. It’s the foundation for constructing AI algorithms, enabling effective extraction of insights and patterns from data, and empowering intelligent decision-making. In this article, we will delve into the core principles and techniques that underpin feature engineering in ML. Additionally, we will explore feature engineering in NLP and feature engineering in data analytics. Moreover, we will also shed light on the vital role of Feature Engineering Automation in various AI applications. Furthermore, we will see the importance of Feature engineering. Consequently, it transcends data preparation, evolving into the craft of shaping raw data into meaningful, informative representations of actionable value.
Feature Engineering in ML
Machine learning algorithms tirelessly process extensive data, sifting for patterns, enabling predictions, and automating decision-making with remarkable efficiency. However, the effectiveness of these algorithms heavily relies on the quality of the features provided to them. This is where Feature Engineering in ML assumes paramount importance. It is the art and science of crafting and selecting the most informative and relevant features from raw data. This meticulous refinement guarantees that the algorithms possess the optimal information for precise predictions.
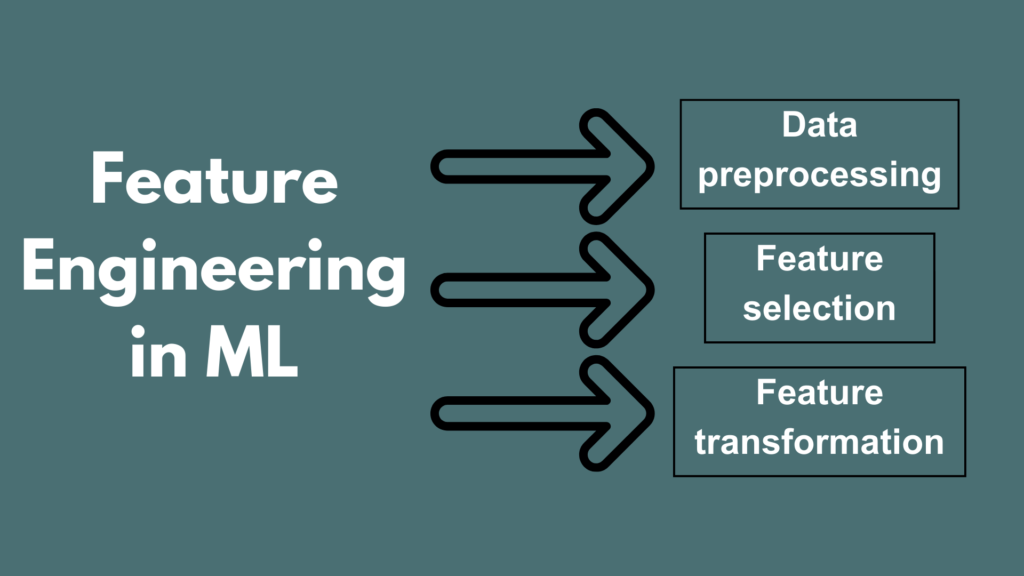
Additionally, feature engineering encompasses various stages, including data preprocessing, feature selection, and feature transformation. To start, data preprocessing includes activities like handling missing values, scaling, and normalization. Subsequently, feature selection aims to pick the most relevant features, eliminating noise and reducing dimensionality. Lastly, feature transformation may involve encoding categorical variables, creating new features, or applying mathematical transformations. These interconnected stages collectively refine the data, enhancing the overall performance of machine learning models.
Feature Engineering in NLP
Natural language processing (NLP) is an AI subfield dedicated to understanding and generating human language. In NLP, text data serves as the primary input. Feature Engineering in NLP is the linchpin that enables machines to make sense of this unstructured information. ML handles structured data effortlessly, whereas NLP grapples with the intricate web of words, sentences, and paragraphs. In NLP, feature engineering involves converting text data into a format digestible by ML algorithms, enabling insightful analysis and understanding.
Feature Engineering Automation
Feature engineering can be an intricate and time-consuming task, often requiring domain knowledge and a creative touch. However, the advent of automation has revolutionized this domain. Feature engineering automation leverages algorithms and tools to streamline the process, making it more efficient and accessible.
One notable platform promoting automated feature engineering is DataRobot. It offers an AI-driven automated machine learning platform that includes feature engineering capabilities. Moreover, it automates the entire machine learning pipeline, handling data preprocessing, and assisting in model selection. Furthermore, this simplifies predictive model creation with minimal manual effort. It’s a powerful tool for automating the entire data-to-insights process.
Feature Engineering in Data Analytics
In the realm of data analytics, the raw material is an abundance of data. Within this domain, Feature Engineering in Data Analytics plays a pivotal role in unveiling insights and trends. The process entails selecting and transforming raw dataset variables, tailoring them to suit the specific analytical task at hand effectively.
Furthermore, feature engineering in data analytics extends beyond model improvement; it focuses on extracting invaluable insights crucial for business decisions. Moreover, whether it’s analyzing sales data, customer behavior, or market trends, the quality of features impacts the quality of conclusions.
Feature Engineering in Time Series
Time series data is ubiquitous, and found in applications ranging from financial forecasting to environmental monitoring. Additionally, in feature engineering time series, the temporal aspect of the data takes center stage. Unlike static data, time series data has a sequential nature, where observations are ordered by time.
Furthermore, feature engineering for time series data involves the creation of lag features, rolling statistics, and time-based aggregations. These engineered features capture the temporal dependencies and patterns within the data, allowing ML algorithms to make accurate predictions. Notably, time series forecasting, stock price prediction, and demand forecasting are just a few examples of where this practice proves invaluable.
Automated Feature Engineering in Kaggle
Kaggle, the popular platform for data science competitions, offers a wealth of tools and resources for data scientists. Notably, among them, automated feature engineering in Kaggle has emerged as a valuable resource. Kaggle’s feature engineering tools and libraries allow data scientists to expedite the feature engineering process.
Furthermore, by leveraging Kaggle’s automated feature engineering capabilities, data scientists can significantly cut down the time spent on data preparation. Consequently, this frees up more time for them to concentrate on experimentation and refining their models. As a result, Kaggle’s user-friendly environment has democratized feature engineering and made it accessible to a community of data enthusiasts.
The Importance of Feature Engineering
The importance of feature engineering extends beyond the individual domains of ML, NLP, or data analytics. Furthermore, it serves as a hidden driver that fuels AI success across the board. Well-engineered features can make the difference between a model that barely scrapes by and one that excels.
Moreover, effective feature engineering can lead to improved model accuracy, reduced overfitting, and faster model convergence. Additionally, it can enhance model interpretability, aiding stakeholder trust and comprehension of AI-driven decisions. Notably, interpretable models foster confidence, bolstering the acceptance of AI systems in various industries and applications.
Road Ahead
The future of feature engineering promises exciting developments. Furthermore, automation will continue to play a significant role, with advanced algorithms and tools streamlining the process further. Additionally, we can anticipate increased integration with artificial intelligence, where AI itself assists in feature selection and transformation. The evolution of feature engineering is expected to continue, extending its capabilities to accommodate more complex data types. These may include unstructured and multimedia data, enabling AI to extract meaningful information from a broader range of sources. Moreover, feature engineering will be central in tackling challenges like privacy and bias, ensuring that AI systems are fair and secure. In the road ahead, feature engineering will remain an indispensable component in the ever-advancing landscape of AI.
In conclusion, feature engineering is the unsung hero behind the remarkable advancements in artificial intelligence. Moreover, it transcends the boundaries of machine learning, natural language processing, data analytics, and time series analysis. This establishes a foundation for insightful decision-making. As automation continues to reshape this field, the future of feature engineering promises even greater efficiency and adaptability. Additionally, its significance extends beyond the technical realms, with profound implications for businesses and industries. So, join us on this adventure, where feature engineering is the compass guiding us toward a brighter, more intelligent world. The future is here, and it’s brimming with promise and potential.
One Response